AI Is a Key to Data-Driven HR, part 1
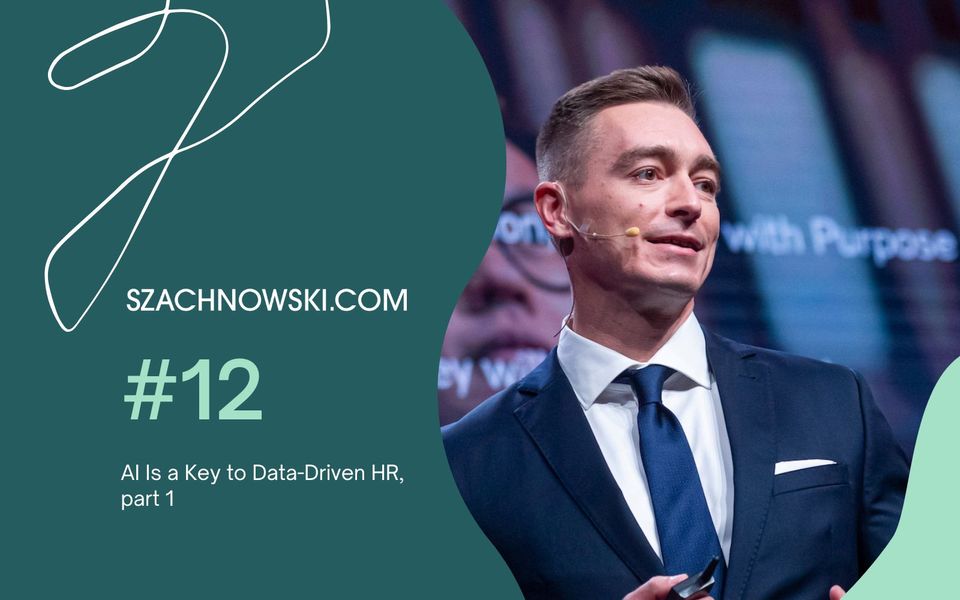
First of all, I’d like to warm welcome all new subscribers of my newsletter! Within the last two weeks the total number of subscribers has increased by 48% and the counter stopped at 128. Thank you for your trust. I hope you will find many interesting topics in the newsletter and multiple opportunities to learn from the content I publish.
Because of so many new readers, I will briefly introduce myself here. My name is Sebastian Szachnowski, I work at Volvo Group since 2007. I live in Wroclaw, Poland. I have quite unique experience and expertise from both HR and IT fields. Therefore, I write about data- and technology-driven HR. My goal is to share knowledge about modern, digital, and innovative approach to HR, but also to promote science in HR. Just recently, I spoke with a colleague, and she mentioned that nobody likes to listen or read about science in HR. Yet, I’ll try anyway.
This week I planned to write about two topics: generative AI impact on HR, and data literacy in HR. However, when I started to write the introduction of the first subject, it turned out that it will be a very long article. Therefore, to respect your time as a reader, I’ll divide the article into two parts. This week, I publish part one, and in one of the following issues, I’ll publish part two where I will elaborate more on practical implementation of generative AI in HR.
Next week, I’ll be present at People Analytics World in London. Will I meet there any of you?
Is AI-Driven HR Just Around The Corner?
Recently, I eventually had time to do some reading and, more importantly, to think through the generic AI revolution and its impact on HR. I’m sure that this technology will be a massive step forward in business. Soon, generative AI will become an essential tool. To get convinced that it will happen, it’s enough to see the recent Microsoft event where they introduced Microsoft 365 Co-pilot that will revolutionize the way we interact with office applications.
What would the generic AI revolution mean to workforce and HR in practice? I’ll try to answer to this question below.
Is Your Work Going to be Automated?
Josh Bersin, well known HR industry analyst, researcher, and thought leader is very enthusiastic about generative HR. He thinks that it will revolutionize business including HR.
Today, as I touch on above, we make thousands of critical decisions with poor data, uneducated judgement, and often just not enough internal research. I believe Generative AI and all its variations will be a total gamechanger in HR. And for everything we do just a little bit better, our employees end up with a better work experience and our companies perform at a higher level.
However, every technology revolution opens a debate regarding its impact on the employment. In other words, what will be the impact of technical progress on automation and future of jobs.
Generative AI appearance once again opened a discussion regarding job automation. Job automation is present in mass media since at least ten years. After 2010, information technology was rapidly developing thanks to decreasing prices of digital storage, increasing computing power, and growth of such services as for example cloud computing. In 2013, Oxford scientists Professor Michael Osborne and Dr Carl Benedikt Frey published a paper “The Future of Employment: How Susceptible Are Jobs To Computerization?”. Since that time, the article was cited almost everywhere in the world and was often taken as a prophecy of inevitable employment apocalypse.
The Oxford researchers suggested that 47% of jobs are automatable from a technological capabilities point of view. Their intention was to show that the scope of jobs automation is vast. They even thought that the 47% of jobs is moderate result. Considering for example that in 1900 over 40% of the workforce was employed in agriculture and now it is less than 2%, through the lens of twentieth century the 47% of jobs exposed to future automation doesn’t seem very high.
We focus on estimating the share of employment that can potentially be substituted by computer capital, from a technological capabilities point of view, over some unspecified number of years. We make no attempt to estimate how many jobs will actually be automated. The actual extent and pace of computerisation will depend on several additional factors which were left unaccounted for.
The study was just an estimate of how exposed existing jobs are to recent developments in artificial intelligence and mobile robotics. It said nothing about the pace at which jobs will be automated. Anyway, it lit a fire and created panic. Newspapers were publishing apocalyptic articles, authors were writing books about end of jobs, and governments quickly started discussions and some tests regarding universal basic income. Societies were preparing for big change that actually never (so far) took place.
This is fact that routine, rule-based activities which are easy to specify in computer code are to very high extend automatable or even automated. Now, the same is happening to another group of jobs that require creativity, content generation, and information synthesis. Again, computers are better than humans in all types of repetitive jobs. There is one prerequisite, however. The job had to be done by humans in the past. Computers still need data to be programmed to fulfil even simple rule-based jobs. Their creativity is also a delusion as they are limited to existing information, and they generate ideas and synthesis of what was previously ideated by humans.
Predictions regarding the future of work are so difficult because they aren’t limited to technical capabilities of computers and robots. Heavy impact on the field have cost of technology, legislation, especially data privacy and compliance, even economics and unknown unknowns, how I like to call unnamed risks like global pandemic that changed the world withing just 2 years.
How difficult it is to predict the future of work was shown on an example of mentioned Oxford’s scientists. In their paper on of jobs that couldn’t be automated according to the study was a waiter. However, in 2016 Eatsa, the very first waiter-less and cashier-less restaurant was opened. I’m not going to elaborate about business model of such place. Yet, it’s an example of how little we know about the future.
During the time when famous Oxford paper was published, a group of researchers at the University of Mannheim did similar study and suggested that only 9% of jobs are exposed to automation. Where this difference comes from?
Every occupation employs thousands of individuals, of which some potentially perform slightly different tasks. This was also the point that the authors of the Mannheim as well as the OECD study picked up on, although within-occupation variation probably plays a minor role: the job of a taxi driver or a cashier, for example, is essentially the same across companies and locations. Still, by using individual instead of occupational level data, allowing them to also capture within-occupation variation, this approach has its merits.
Researchers from the University of Mannheim studied not only jobs and task, but also demographic variables such as sex, education, age, and income.
According to this approach, the more an accountant earns, the less automatable his job is. If he or she happens to have a PhD in sociology, the job is safer from automation. Similarly, a female taxi driver with a PhD is less likely to be displaced by a self-driving car than a man who has been driving a taxi for decades.
Forecasting future is tough. Policymakers will have to understand not only information coming from the research, but also thinking behind, to draw their own conclusions, assess the scale, and craft appropriate responses.
Deconstructing Jobs into Work Elements
World of work has that tendency to offset automation and technical progress with increased productivity and new jobs. However, as Nassim Nicholas Taleb describes in his book “Fooled by Randomness”, we shouldn’t be overconfident regarding our capabilities to understand probability and estimation of likelihood of the occurrence of rare events. It is important to remain humble and skeptical of those who claim to have all answers. Past performance is not reliable predictor of the future. We need to be prepared to unexpected events and be able to adapt quickly to changing circumstances.
Current unemployment rate in EU is at very low level. In January 2023 it was 6.1% for all 27 countries. In the US in January 2023 was 50-years low 3.4%. All this despite those apocalyptic prophecies about 47% of jobs to be automated. I demystified the apocalyptic visions distributed by media and people, but the automation is a fact. Recent years we automated a lot of work. We didn’t reduced the number of jobs, however.
In their book “Reinventing Jobs”, Ravin Jesuthasan and John W. Boudreau brought an example of ATM. How many bank teller jobs can be replaced by ATMs? In the 1970s, if you’re bold enough, you could even plan to close number of branches of banks and fully replace them with mini-branches consisting solely of ATMs.
That didn’t happen, though. But why?
Technology, despite of increasing efficiency, usually is sold by presenting how it will reduce risk, reduce mistakes, increase scalability, reduce lead time, and so forth. Technology is never a revolution, it’s an enabler to a better solution.
The first step to better solution is to deconstruct the job into tasks.
Example of bank teller job might look like below:
- Greeting and welcoming customers
- Receiving customer’s request for cash withdrawal
- Verifying that customer’s account balance contains sufficient funds
- Processing the withdrawal to debit the customer’s checking account
- Counting and giving cash to the customer
- Counselling customers when account balances are insufficient to process the transaction
- Engaging the customers in conversation
- Detecting customer’s receptivity to additional banking services
- Recommending and describing additional banking services
- Referring customers to other bank employees for further services and products
- Collaborating with bank product designers and process leaders to improve products and processes
Some tasks are easy to automate with ATMs. Some, like counselling customers are not amenable to automation. ATM can hardly deal with customers emotions and sentiments.
The ATM example shows that we must deconstruct jobs into their key elements and not think in terms of replacing entire jobs.
That does not mean that jobs will disappear, but rather that they will be reinvented, as work that was aggregated into a “job” is constantly reconfigured and continuously deconstructed and reconstructed. Over time, some work elements will be removed from the job as they are transferred to other work arrangements or automation.
In practice that means that every time we make technological progress, and we automate rule-based or cognitive tasks, we also create new jobs, tasks, in other words we leverage the progress to innovate, be more effective, grow global economy and create new jobs that offsets these that were automated. However, event trusting this pattern we must remain humble and remember that past performance is not reliable predictor of the future.
Generative AI Revolution
Let’s start with description what generative AI is not. Generative AI is not AGI which opens to Artificial General Intelligence. Holy grail of researchers, the one we know from sci-fi novels and movies. Singularity that is smart and often malicious like Hal 9000 or Skynet.
Generative AI is type of artificial intelligence that is designed to generate content such as text, images, or videos. It uses deep learning neural networks to learn from patterns and similarities in data, and then use that learning to create new data. In other words, it generates output out of learning data it was fed with. Generative AI models typically require large amount of data to learn, and are trained using techniques such as unsupervised learning, reinforcement learning, or adversarial training.
However, it is a big AI revolution. We used to utilize machine learning and deep learning for quite a while. A lot of everyday services use big data to create value for end users. Companies use data and AI to learn about their users and customers. We get recommendations of products or services based on AI predictions. However, due to their limitations these models are often referred as narrow or analytical AI.
It changed since November 2022. Until then, there was no technology capable of reinventing user interfaces and human-computer interactions. Now, that kind of technology is available, and if applied in hybrid human-machine configuration, it can strongly enhance creativity, productivity and efficiency of people. It has both professional and personal applications.
Moving back to the jobs automation context, Goldman Sachs published a report: “The Potentially Large Effects of Artificial Intelligence on Economic Growth (Briggs/Kodnani)”.
If generative AI delivers on its promised capabilities, the labor market could face significant disruption. Using data on occupational tasks in both the US and Europe, we find that roughly two-thirds of current jobs are exposed to some degree of AI automation, and that generative AI could substitute up to one-fourth of current work. Extrapolating our estimates globally suggests that generative AI could expose the equivalent of 300mn full-time jobs to automation.
They are quite optimistic regarding prospective worker displacement being offset by creation of new jobs.
The good news is that worker displacement from automation has historically been offset by creation of new jobs, and the emergence of new occupations following technological innovations accounts for the vast majority of long-run employment growth. The combination of significant labor cost savings, new job creation, and higher productivity for non-displaced workers raises the possibility of a productivity boom that raises economic growth substantially, although the timing of such a boom is hard to predict.
According to the researchers, the AI caused boost in productivity should be economically significant.
The boost to global labor productivity could also be economically significant, and we estimate that AI could eventually increase annual global GDP by 7%. Although the impact of AI will ultimately depend on its capability and adoption timeline, this estimate highlights the enormous economic potential of generative AI if it delivers on its promise.
However, Goldman Sachs researchers state that although the impact of AI on the labor market and economy will be significant, most jobs and industries are only partially exposed to automation. They are more likely to be complemented (in a hybrid human-machine model) than substituted by AI.
Historically, the impact of technology innovations introduced new occupations, like software developers, data scientists, digital marketers, but also increased aggregated income and directly drove demand for service sector workers and industries such like health care, education, and food services. In the past, technology innovations displaced workers but also created new employment opportunities at roughly the same rate.
Generative AI Impact on HR
Workforce cost – including but not limited to salaries – is one of the highest cost elements of all corporate spendings. Yet, Human Resources remains complex but imperfect area of business. That imperfection is visible in judgmental decisions made regarding the workforce. These decisions are usually based on experience and intuition and are highly biased. These biases cost companies a lot of money.
We put a lot of effort to introduce informed decision making in HR. We are constantly developing practices of people analytics and we transform our HR departments into data-driven corporate functions.
Josh Bersin notices that a lot of HR data is text data we collect from resumes, work output, assessments, and communications. The ability to process that data in traditional manner is limited.
So if you want to really do a “big data” analysis of your workforce’s skills, experience, and suitability for different work, you’re dealing with mountains of “anecdotal data,” much of which is encoded in biographies, work output, company leadership frameworks, assessments, and lots of communications. And of course, there are performance appraisals, business results, and more.
Adequately trained generative AIs, and Large Language Models (LLM) in particular, come to solve that problem and enable the next level prescriptive analytics based on HR data. But more on that in the next issue.
Sources:
- https://www.oxfordmartin.ox.ac.uk/blog/automation-and-the-future-of-work-understanding-the-numbers/
- https://www.oxfordmartin.ox.ac.uk/downloads/academic/future-of-employment.pdf
- https://mashable.com/article/eatsa-waiterless-cashierless-tech-restaurant
- https://joshbersin.com/2023/03/the-role-of-generative-ai-and-large-language-models-in-hr/
- The Potentially Large Effects of Artificial Intelligence on Economic Growth (Briggs/Kodnani)
Inspiring quote
“A diamond is merely a lump of coal that did well under pressure.” — Henry Kissenger
Want to be a Sponsor?
Would you like to be a sponsor of my newsletter? Contact me: sebastian@szachnowski.com.
Thank you for reading my newsletter! I’ll be delighted to get your feedback about the newsletter and this issue.
Do you know someone who might be interested in this newsletter? Share it with them.
You can also:
- Subscribe to my newsletter https://szachnowski.com/
- Connect with me on LinkedIn https://www.linkedin.com/in/sebastianszachnowski/
- Follow me on Twitter https://twitter.com/sszachnowski
- Send me an email sebastian@szachnowski.com